Some of machine learning’s main issues are around time and cost and the amount of training material required to train models that are useful and produce accurate, pleasing results. Why is it so difficult?
AI tools are ‘learning’ not to be caught out by people asking them nonsensical questions and have started to give as good as they get. For example, when somebody asked ChatGPT about messing with a San Francisco icon, its answer was, “There is no record or historical event indicating that the Golden Gate Bridge, which is located in San Francisco, California, USA, was ever transported across Egypt”.
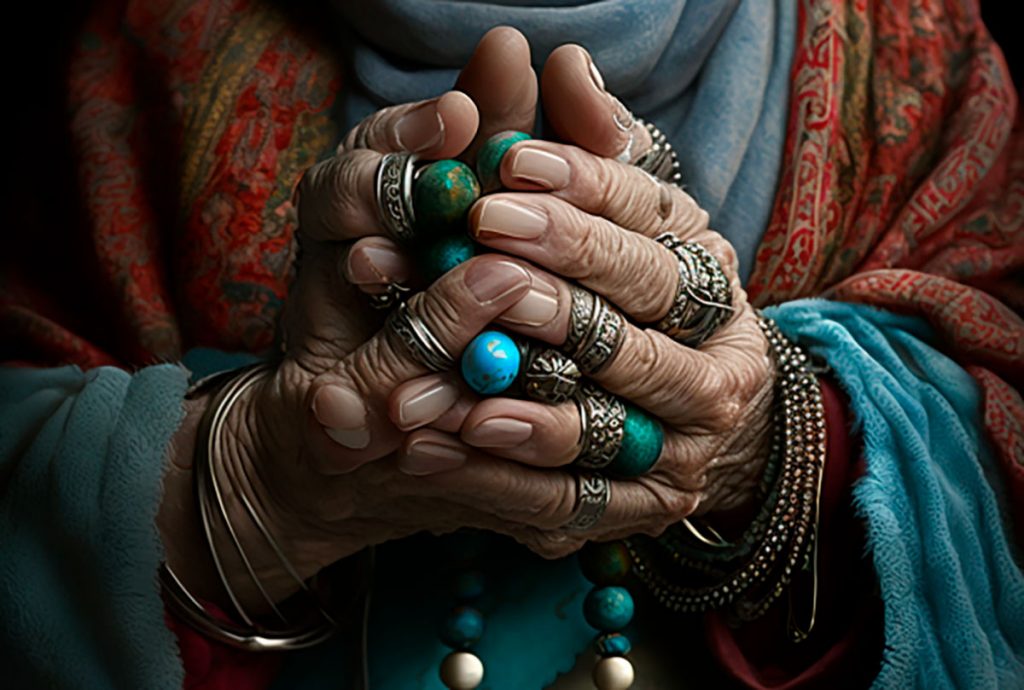
However, when it comes to AI-generated content, particularly visuals, machine learning-based tools still hallucinate and return results that are very obviously wrong, such as historically inaccurate depictions and the ongoing issue with hands. But what causes the hallucinations?
Stefano Soatto, vice president and distinguished scientist at AWS characterises an hallucination in AI as “synthetically generated data” or “fake data that is statistically indistinguishable from actual factually correct data”. For example, the purpose of an AI model that can generate text and was trained on Wikipedia is to generate text that is “statistically indistinguishable” from the training data (Wikipedia). It’s all about how the machine learning model was trained and the amount and quality of training material. A machine learning model doesn’t possess context. It has to be drummed into it through repetitive, consistent exposure to contextually correct information.
“If users hope to download a pre-trained model from the web and just run it and hope that they get factual answers to questions, that is not a wise use of the model because that model is not designed and trained to do that.
But if they use services that place the model inside a bigger system where they can specify or customize their constraints … that system overall should not hallucinate.”
Stefano Soatto, vice president and distinguished scientist, Amazon Web Services
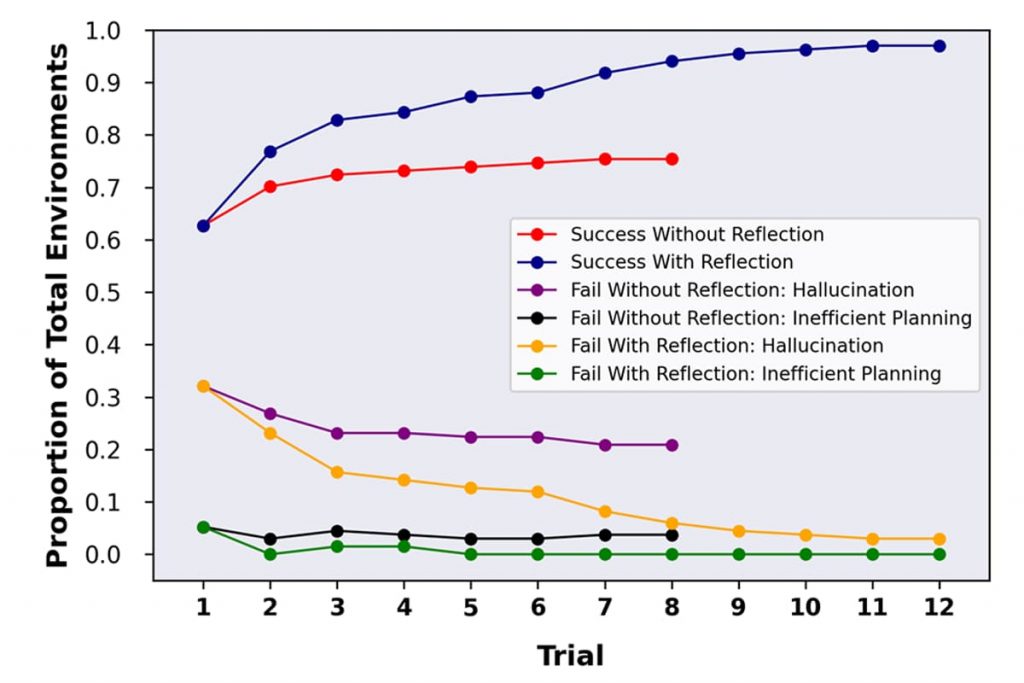
Some of the platforms warn users about this and reassure them that they’re getting better; OpenAI say that GPT-4, which was released in March 2023 “is 40% more likely” to produce factual responses than its predecessor, GPT-3.5. Independent verification of results, checking consistency and “factuality” and tracing attribution are the route to a more accurate future.
“I think it helps to not expect of machines what even humans cannot do, especially when it comes to interpreting the intent of humans. It’s important for humans to understand [AI models], exploit them for what they can do, mitigate the risks for what they’re not designed to do and design systems that manage them.”
Stefano Soatto, vice president and distinguished scientist, Amazon Web Services
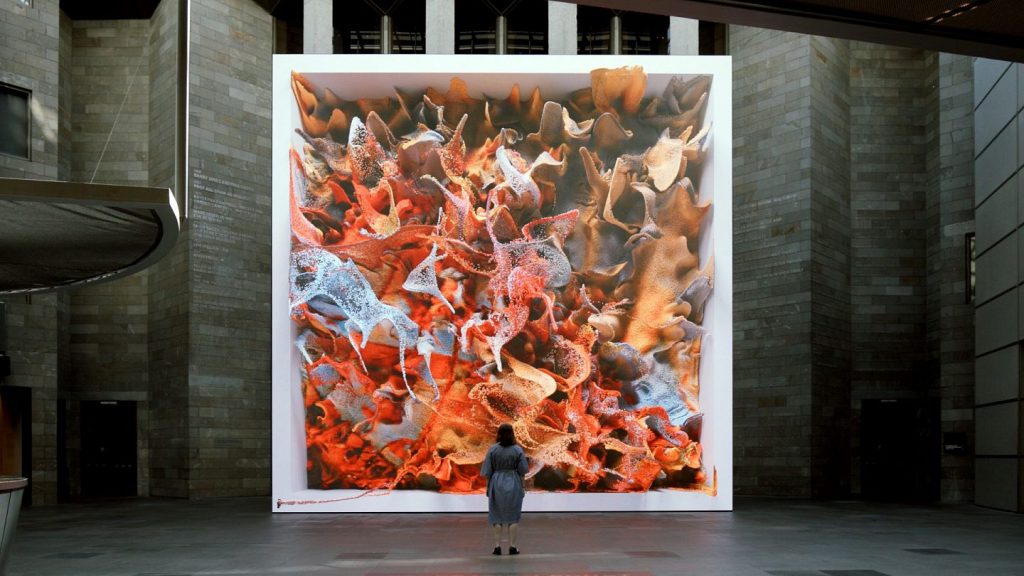
Hallucinations can be a good thing, too, as they produce results that might not occur to a human and some models are trained, or trained with fewer constraints, in order to achieve the fantastical or the unexpected. The work of artist Refik Anadol is a good example of this.
Hallucination in generative AI will dissipate over time, as more training material is thrown at the models. Transparency, accountability and human oversight will also play a big part. In the meantime, more care and attention will help, but hallucination and inaccuracy will remain an annoying or inspiring feature of machine learning.
For advice, guidance and support on how to safely and reliably incorporate machine learning into your creative and production processes and workflows, Mondatum is here to help. Your first points of contact are Colin Birch (colin@mondatum.com) and John Rowe (john@mondatum.com).
Source: CNET