Those who follow The Beautiful Game will be very familiar with the ugly scenes, in stands, living rooms and pubs as well as on the pitch, when a contentious offside decision goes to VAR and some nonsense occurs. The Euros gave us hope, when we saw incontestable instant decision-making in top-flight football for the first time.
A new semi-automated offside system code-named Dragon from Genius Sports and subsidiary Second Spectrum, who made their name in the NBA, and which is powered by “dozens” of iPhones (iPhone 14 or newer), is expected to be introduced some time later in the 2024–25 English Premiership season. It captures a constant stream of high frame-rate video from multiple angles and processes them into a single unified multi-dimensional view.
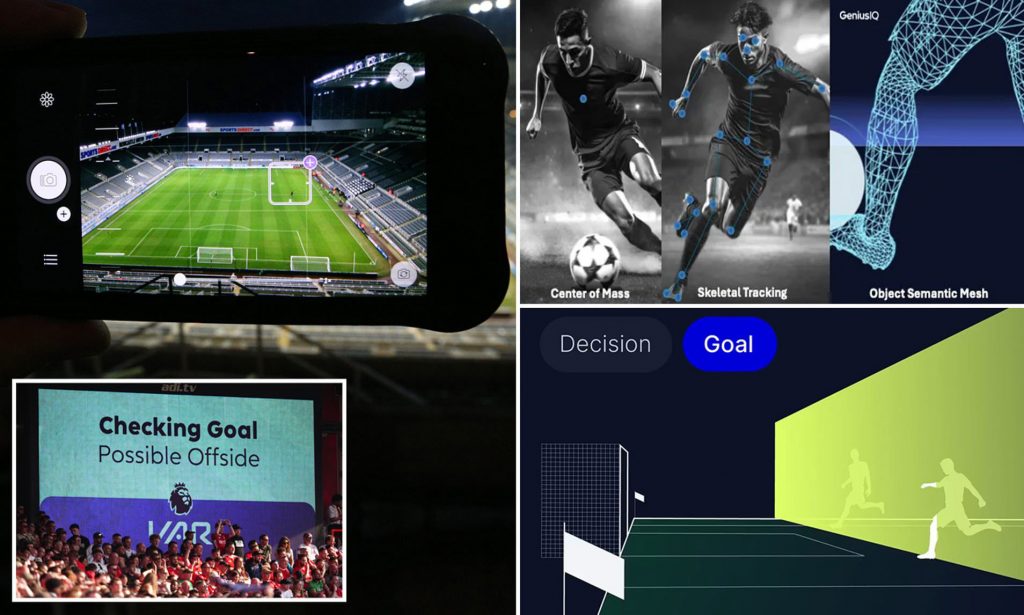
The problem with this type of technology has always been occlusion. Ben Dowsett, writing in Wired and here referring to the NBA.
Even with a dozen or so cameras, the right angle isn’t always visible to view entire plays. Historically, machine learning systems have filled in those gaps with what amounts to educated guesses about where uncaptured elements are likely to be. For many use cases, those types of guesses are fine. But once you’re in a situation where guessing isn’t good enough, like when game referees are relying on your technology to adjudicate a call on the field, a higher standard of performance has to be enforced.
Ben Duckett, Wired
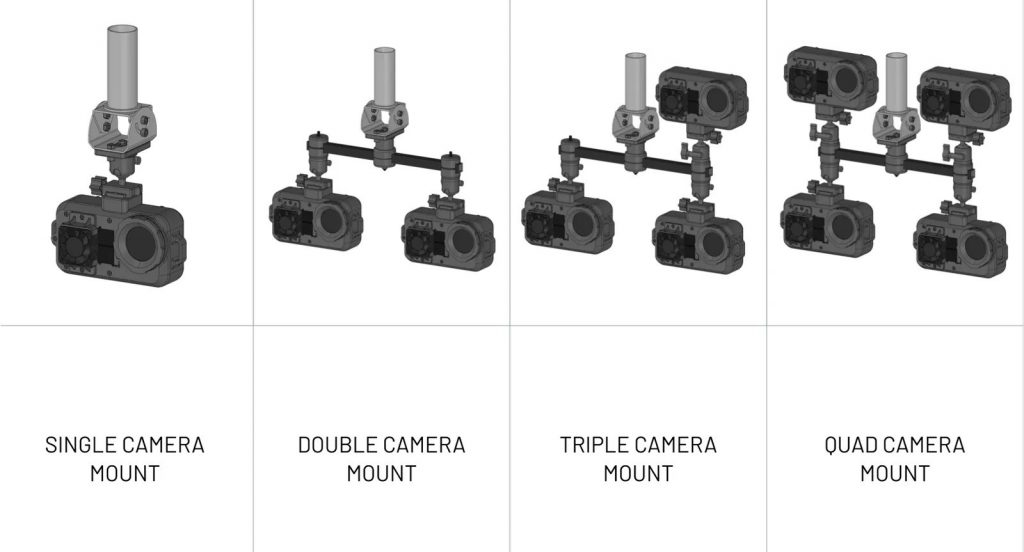
The system we have seen in use in the Premier League most recently uses “between 10 and 15 cameras and a sensor inside the ball to track a few dozen body points on each player. But they too often fall victim to occlusion and can’t accurately parse these fleeting moments”. Dragon “will initially use at least 28 iPhone cameras at every stadium in the Premier League” and “track between 7,000 and 10,000 points on every player at all times” at, initially, 100 frames per second. Every relevant type of physical metric and positional reference imaginable is captured to create a true digital duplicate of the actual scene.
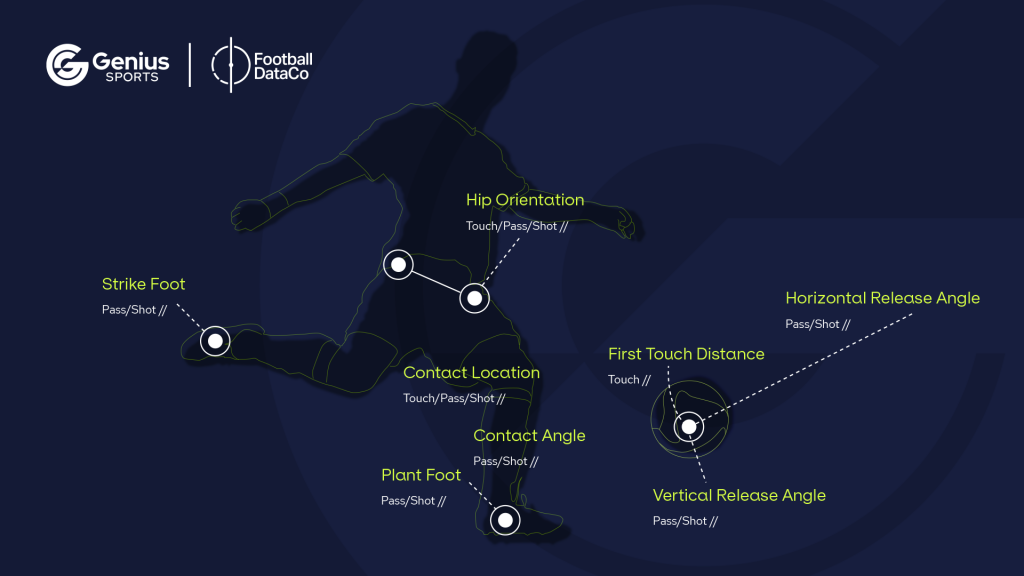
The system is also intelligent. It can anticipate an event occurring and increase resolution dynamically to improve accuracy. A machine learning model known internally as the “object semantic mesh” is trained on videos depicting common events and occurrences in football matches. “It’s not just capturing movements, it’s contextualizing them in real time—and in some cases even learning from them”.
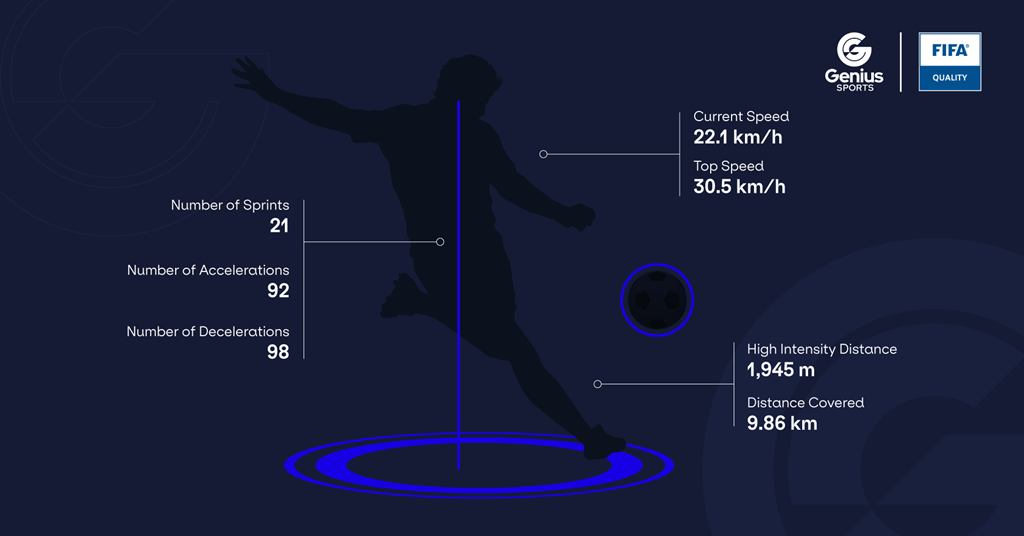
At this point, the prospect of a sports entertainment brand getting its hands on digital twins of football matches begins to emerge, and watching the game on a VR headset takes another step towards becoming a reality.