A team of researchers at Duke University in the US led by computer scientists Cynthia Rudin has successfully used machine learning to convert blurry portrait photos containing just a few dozen pixels into a super high-resolution images.
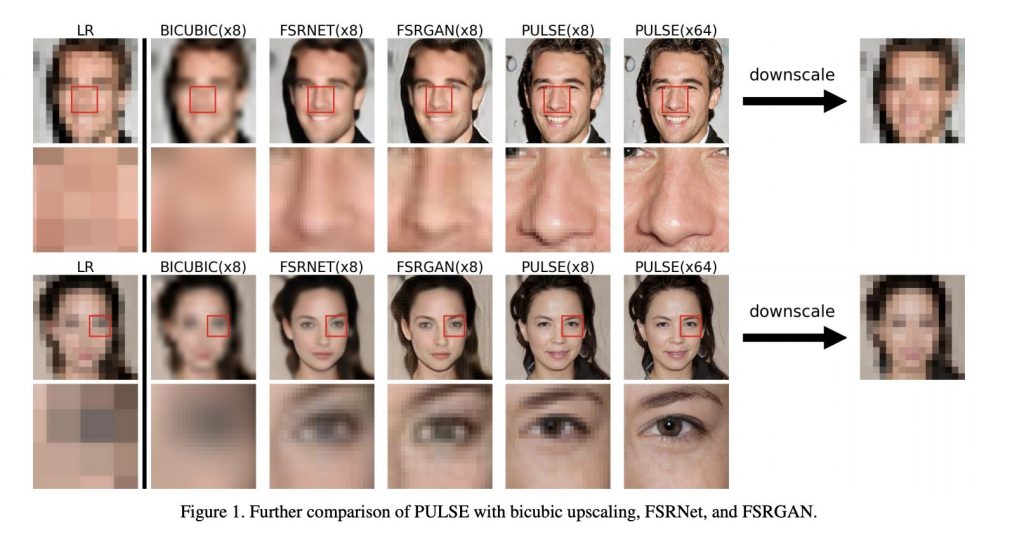
The PULSE (Photo Upsampling via Latent Space Exploration) system works by reverse engineering the image from AI-generated high resolution images that look similar to the low resolution image when downscaled and can enhance the picture by up to 64-times its original resolution.
Traditional approaches take a low-resolution image and ‘guess’ what extra pixels are needed by trying to get them to match, on average, with corresponding pixels in high-resolution images the computer has seen before, but textured areas in hair and skin that might not line up perfectly from one pixel to the next end up looking fuzzy and indistinct.
Instead of taking a low-resolution image and slowly adding new detail, PULSE searches through AI-generated examples of high-resolution faces to find examples that match the input image when reduced to the same size.
PULSE uses a GAN (generative adversarial network), where two neural networks are trained on the same data set of photos. One finds AI-created human faces that are similar to the faces it was trained on. The other decides is this output is accurate enough to be mistaken for the real thing. The first network gets better and better with experience, until the second network can’t tell the difference. PULSE can convert a 16 x 16-pixel image of a face into 1024 x 1024 pixels in a matter of seconds, adding more than a million pixels.
In Cynthia’s words, their algorithm “imagines” what the picture should look like, so the results cannot be used, for example, as part of a CCTV-based facial recognition system.
The team’s research is being presented at this week’s (virtual) 2020 Conference on Computer Vision and Pattern Recognition (CVPR).